Key Concepts
Processes for formulating and disseminating information about future weather conditions based upon the collection and analysis of meteorological observations. Weather forecasts may be classified according to the space and time scale of the predicted phenomena (Fig. 1). Atmospheric fluctuations with a length of less than 100 m (330 ft) and a period of less than 100 s are considered to be turbulent. Prediction of turbulence extends only to establishing its statistical properties, insofar as these are determined by the thermal and dynamic stability of the air and by the aerodynamic roughness of the underlying surface. The study of atmospheric turbulence is called micrometeorology; it is of importance for understanding the diffusion of air pollutants and other aspects of the climate near the ground. Standard meteorological observations are made with sampling techniques that filter out the influence of turbulence. Common terminology distinguishes among three classes of phenomena with a scale that is larger than the turbulent microscale: the mesoscale, synoptic scale, and planetary scale.
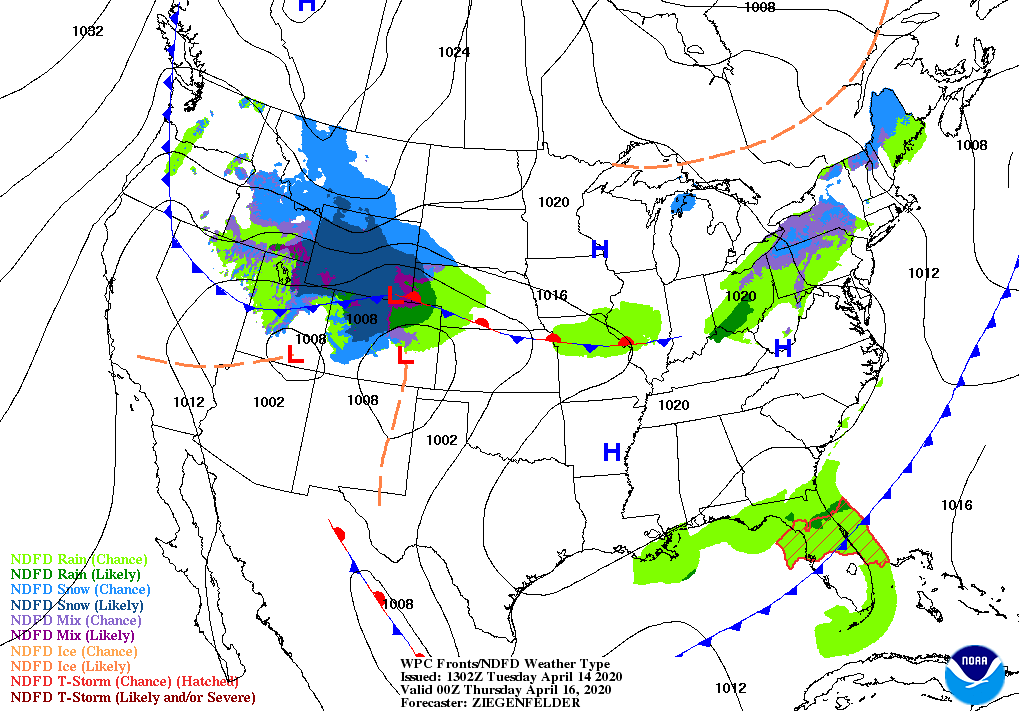
The mesoscale includes all moist convection phenomena, ranging from individual cloud cells up to the convective cloud complexes associated with prefrontal squall lines, tropical storms, and the intertropical convergence zone. Also included among mesoscale phenomena are the sea breeze, mountain valley circulations, and the detailed structure of frontal inversions. Because most mesoscale phenomena have time periods less than 12 h, they are little influenced by the rotation of the Earth. The prediction of mesoscale phenomena is an area of active research. Most forecasting methods depend upon empirical rules or the short-range extrapolation of current observations, particularly those provided by radar and geostationary satellites. Forecasts are usually couched in probabilistic terms to reflect the sporadic character of the phenomena. Since many mesoscale phenomena pose serious threats to life and property, it is the practice to issue advisories of potential occurrence significantly in advance. These “watch” advisories encourage the public to attain a degree of readiness appropriate to the potential hazard. Once the phenomenon is considered to be imminent, the advisory is changed to a “warning,” with the expectation that the public will take immediate action to prevent the loss of life.
The next-largest scale of weather events is called the synoptic scale, because the network of meteorological stations making simultaneous, or synoptic, observations serves to define the phenomena. The migratory storm systems of the extratropics are synoptic-scale events, as are the undulating wind currents of the upper-air circulation which accompany the storms. The storms are associated with barometric minima, variously called lows, depressions, or cyclones. The sense of the wind rotation about the storm is counterclockwise in the Northern Hemisphere, but clockwise in the Southern Hemisphere. This effect, called geostrophy, is due to the rotation of the Earth and the relatively long period, 3–7 days, of the storm life cycle. Significant progress has been made in the numerical prediction of synoptic-scale phenomena.
Planetary-scale phenomena are persistent, quasi-stationary perturbations of the global circulation of the air with horizontal dimensions comparable to the radius of the Earth. These dominant features of the general circulation appear to be correlated with the major orographic features of the globe and with the latent and sensible heat sources provided by the oceans. They tend to control the paths followed by the synoptic-scale storms, and to draw upon the synoptic transients for an additional source of heat and momentum. Long-range weather forecasts must account for the slow evolution of the planetary-scale circulations. To the extent that the planetary-scale centers of action can be correctly predicted, the path and frequency of migratory storm systems can be estimated. The problem of long-range forecasting blends into the question of climate variation. See also: Atmosphere; Meteorological instrumentation; Micrometeorology; Weather observations
The synoptic method of forecasting consists of the simultaneous collection of weather observations, and the plotting and analysis of these data on geographical maps (Fig. 1). An experienced analyst, having studied several of these maps in chronological succession, can follow the movement and intensification of weather systems and forecast their positions. This forecasting technique requires the regular and frequent use of large networks of data. See also: Weather map
The synoptic forecasts are limited because they are almost exclusively based upon surface observations. The stratosphere, a layer of air generally located above levels from 9 to 13 km (5.5 to 8 mi) and characterized by temperatures remaining constant or increasing with elevation, acts as a cap on much of the world's weather. A trackable instrument suspended beneath a rubber balloon (the radiosonde) is routinely deployed from a network of stations. The tracking of the balloon allows for wind measurements to be taken at various elevations throughout its ascent. Such a network of more than 2000 upper-air stations around the world reports its observations twice daily. Satellite measurements and aircraft observations supplement the radiosonde network and are utilized to improve the analysis and tracking of weather systems.
The basic laws of hydrodynamics and thermodynamics can be used to define the current state of the atmosphere, and to compute its future state. This concept marked the birth of the dynamic method of forecasting, though its practical application was realized only after 1950, when sufficiently powerful computers became available to produce the necessary calculations efficiently. The first conceptual model of the life cycle of a surface cyclone showed that cyclonic storms typically formed along fronts, that is, boundaries between cold and warm air masses. The model showed that precipitation is associated with active cold and warm fronts, and that this precipitation wraps around the cyclone as it intensifies. Though subsequent work demonstrated that fronts are not crucial to cyclogenesis, the Norwegian frontal cyclone model is still used in weather map analyses. See also: Cyclone; Front
It was not until the late 1930s that routine aerological observations had a substantial impact on forecasting. By the 1950s, qualitatively reasonable numerical forecasts of the atmosphere were produced. Numerical weather prediction techniques, in addition to being applied to short-range weather prediction, are used in such research studies as air-pollutant transport and the effects of greenhouse gases on global climate change. See also: Air pollution; Greenhouse effect; Jet stream; Upper-atmosphere dynamics
Though numerical forecasts continue to improve, statistical forecast techniques, once used exclusively with observational data available at the time of the forecast, are now used in conjunction with numerical output to predict the weather. Statistical methods, based upon a historical comparison of actual weather conditions with large samples of output from the same numerical model, routinely play a role in the prediction of surface temperatures and precipitation probabilities.
The recognition that small, barely detectable differences in the initial analysis of a forecast model often lead to very large errors in a 12–48-h forecast has led to experiments with ensemble forecasting. This method uses results from several numerical forecasts to produce the statistical mean and standard deviation of the forecasts. Success with ensemble forecasting suggests it can be a useful tool in enhancing prediction skill and in assessing the atmosphere's predictability. See also: Dynamic meteorology
The best weather forecasts result from application of the synoptic method to the latest numerical and statistical information. The forecaster has an ever-increasing number of valuable tools with which to work. Numerical forecast models, owing to faster computers, are capable of explicitly resolving mesoscale weather systems. Geostationary satellites allow for continuous tracking of such dangerous weather systems as hurricanes (Fig. 2). The increased routine use of Doppler radar, automated commercial aircraft observations, and use of data derived from wind and temperature profiler soundings promise to give added capability in tracking and forecasting mesoscale weather disturbances. Very high-frequency and ultrahigh-frequency Doppler radars may be used to provide detailed wind soundings. These wind profilers, if located sufficiently close to one another, will allow for the hourly tracking of mesoscale disturbances aloft. Ground- and satellite-based microwave radiometric measurements are being used to construct temperature and moisture soundings of the atmosphere. The assimilation of such data at varying times in the numerical model forecast cycle offers the promise of improved prediction and improved utilization of data and products by forecasters assisted by increasingly powerful interactive computer systems. Medium-range forecasts, ranging up to two weeks, may be improved from knowledge of forecast skill in relationship to the form of the planetary-scale atmospheric circulation. See also: Doppler radar; Mesometeorology; Meteorological radar; Meteorological rocket; Meteorological satellites; Radar meteorology; Satellite meteorology
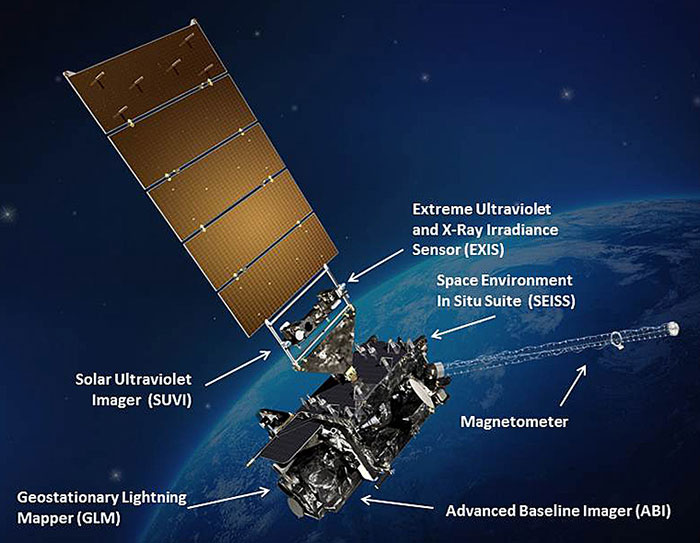
Numerical Weather Prediction
Numerical weather prediction is the prediction of weather phenomena by the numerical solution of the equations governing the motion and changes of condition of the atmosphere.
The laws of motion of the atmosphere may be expressed as a set of partial differential equations relating the temporal rates of change of the meteorological variables to their instantaneous distribution in space. These equations are developed in dynamic meteorology. In principle, a prediction for a finite time interval can be obtained by summing a succession of infinitesimal time changes of the meteorological variables, each of which is determined by their distribution at a given instant of time. However, the nonlinearity of the equations and the complexity and multiplicity of the data make this process impossible in practice. Instead, it is necessary to resort to numerical approximation techniques in which successive changes in the variables are calculated for small but finite time intervals over a domain spanning part or all of the atmosphere. Even so, the amount of computation is vast, and numerical weather prediction remained only a dream until the advent of the modern computer.
The accuracy of numerical weather prediction depends on (1) an understanding of the physical laws of atmospheric behavior; (2) the ability to define through observations and analysis the state of the atmosphere at the initial time of the forecast; and (3) the accuracy with which the solutions of the continuous equations describing the rate of change of atmospheric variables are approximated by numerical means. The greatest success has been achieved in predicting the motion of the large-scale (>1600 km or 1000 mi) pressure systems in the atmosphere for relatively short periods of time (1–5 days). For such space and time scales, the poorly understood energy sources and frictional dissipative forces may be approximated by relatively simple formulations, and rather coarse horizontal resolutions (100–200 km or 60–120 mi) may be used.
Great progress has been made in improving the accuracy of numerical weather prediction models. Forecasts for three, four, and five days have steadily improved. Because of the increasing accuracy of numerical forecasts, numerical models have become the basis for medium-range (1–10 days) forecasts made by the weather services of most countries. See also: Mesometeorology
Cloud and precipitation prediction
If, to the standard dynamic variables, the density of water vapor is added, it becomes possible to predict clouds and precipitation in addition to the air motion. When a parcel of air containing a fixed quantity of water vapor ascends, it expands adiabatically and cools until it becomes saturated. Continued ascent produces clouds and precipitation. The most successful predictions made by this method are obtained in regions of strong rising motion, whether induced by forced orographic ascent or by horizontal convergence in well-developed cyclones. The physics and mechanics of the convective cloud-formation process make the prediction of convective cloud and showery precipitation more difficult.
Global prediction
In 1955, the first operational numerical weather prediction model was introduced at the National Meteorological Center. This simplified barotropic model consisted of only one layer, and therefore it could model only the temporal variation of the mean vertical structure of the atmosphere. By the early 1990s, the speed of computers had increased sufficiently to permit the development of multilevel (usually about 10–20) models that could resolve the vertical variation of the wind, temperature, and moisture. These multilevel models predicted the fundamental meteorological variables for large scales of motion. Global models with horizontal resolutions as fine as 200 km (125 mi) are used by weather services in several countries.
Global numerical weather prediction models require powerful supercomputers to complete a 10-day forecast in a reasonable amount of time. For example, a 10-day forecast with a 15-layer global model with horizontal resolution of 150 km (90 mi) requires approximately 1012 calculations. A supercomputer capable of performing 108 arithmetic operations per second would then require 104 s or 2.8 h to complete a single 10-day forecast. See also: Supercomputer
Numerical models of climate
While global models were being implemented for operational weather prediction 1–10 days in advance, similar research models were being developed that could be applied for climate studies by running for much longer time periods. The extension of numerical predictions to long time intervals (many years) requires a more accurate numerical representation of the energy transfer and turbulent dissipative processes within the atmosphere and at the air-earth boundary, as well as greatly augmented computing-machine speeds and capacities.
With state-of-the-art computers, it is impossible to run global climate models with the same high resolution as numerical weather prediction models; for example, a hundred-year climate simulation with the numerical weather prediction model that requires 2.8 h for a 10-day forecast would require 10,220 h (1.2 years) of computer time. Therefore, climate models must be run at lower horizontal resolutions than numerical weather prediction models (typically 400 km or 250 mi).
Predictions of mean conditions over the large areas resolvable by climate models are feasible because it is possible to incorporate into the prediction equations estimates of the energy sources and sinks—estimates that may be inaccurate in detail but generally correct in the mean. Thus, long-term simulations of climate models with coarse horizontal resolutions have yielded simulations of mean circulations that strongly resemble those of the atmosphere. These simulations have been useful in explaining the principal features of the Earth's climate, even though it is impossible to predict the daily fluctuations of weather for extended periods. Climate models have also been used successfully to explain paleoclimatic variations, and are being applied to predict future changes in the climate induced by changes in the atmospheric composition or characteristics of the Earth's surface due to human activities. See also: Climate history; Climate modification
Limited-area models
Although the relatively coarse grids in global models are necessary for economical reasons, they are sources of two major types of forecast error. First, the truncation errors introduced when the continuous differential equations are replaced with approximations of finite resolution cause erroneous behavior of the scales of motion that are resolved by the models. Second, the neglect of scales of motion too small to be resolved by the mesh (for example, thunderstorms) may cause errors in the larger scales of motion. In an effort to simultaneously reduce both of these errors, models with considerably finer meshes have been tested. However, the price of reducing the mesh has been the necessity of covering smaller domains in order to keep the total computational effort within computer capability. Thus the main operational limited-area model run at the National Meteorological Center has a mesh length of approximately 80 km (50 mi) on a side and covers a limited region approximately two times larger than North America. Because the side boundaries of this model lie in meteorologically active regions, the variables on the boundaries must be updated during the forecast. A typical procedure is to interpolate these required future values on the boundary from a coarse-mesh global model that is run first. Although this method is simple in concept, there are mathematical problems associated with it, including overspecification of some variables on the fine mesh. Nevertheless, limited-area models have made significant improvements in the accuracy of short-range numerical forecasts over the United States.
Even the small mesh sizes of the operational limited-area models are far too coarse to resolve the detailed structure of many important atmospheric phenomena, including hurricanes, thunderstorms, sea- and land-breeze circulations, mountain waves, and a variety of air-pollution phenomena. Considerable effort has gone into developing specialized research models with appropriate mesh sizes to study these and other small-scale systems. Thus, fully three-dimensional hurricane models with mesh sizes of 8 km (5 mi) simulate many of the features of real hurricanes. On even smaller scales, models with horizontal resolutions of a few hundred meters reproduce many of the observed features in the life cycle of thunderstorms and squall lines. It would be misleading, however, to imply that models of these phenomena differ from the large-scale models only in their resolution. In fact, physical processes that are negligible on large scales become important for some of the phenomena on smaller scales. For example, the drag of precipitation on the surrounding air is important in simulating thunderstorms, but not for modeling large scales of motion. Thus the details of precipitation processes, condensation, evaporation, freezing, and melting must be incorporated into realistic cloud models.
In another class of special models, chemical reactions between trace gases are considered. For example, in models of urban photochemical smog, predictive equations for the concentration of oxides of nitrogen, oxygen, ozone, and reactive hydrocarbons are solved. These equations contain transport and diffusion effects by the wind as well as reactions with solar radiation and other gases. Such air-chemistry models become far more complex than atmospheric models as the number of constituent gases and permitted reactions increases. See also: Computer programming; Digital computer; Model theory
Modern weather analysis and forecasting procedures involve (1) the collection of global meteorological surface and upper-air observations in place (for example, ships of opportunity) and remotely sensed (for example, satellite sensors) instrumentation platforms; (2) the preparation of global surface and upper air pressure, temperature, moisture, and wind analyses at frequent time intervals based upon these observations; (3) the solution of a closed set of highly nonlinear equations governing atmospheric dynamical motions by numerical means using the most sophisticated computers to predict the future state of the global atmosphere from the initial conditions specified by the analyses in step 2; (4) the application of statistical procedures such as model output statistics (MOS) to the atmospheric simulations obtained from the dynamical models to predict a wide variety of weather elements of interest to potential users by objective means; and (5) the monitoring of the intervention by experienced human forecasters at appropriate stages in this objective forecasting process to improve the quality and breadth of the forecast information communicated to a wide spectrum of users in the private and public sectors.
Data collection and analysis
Surface meteorological observations are routinely collected from a vast continental data network, with the majority of these observations obtained from the middle latitudes of both hemispheres. Commercial ships of opportunity, military vessels, and moored and drifting buoys provide similar in-place measurements from oceanic regions, although the data density is biased toward the principal global shipping lanes. Information on winds, pressure, temperature, and moisture throughout the troposphere and into the stratosphere is routinely collected from (1) balloon-borne instrumentation packages (radiosonde observations) and commercial and military aircraft which sample the free atmosphere directly; (2) ground-based remote-sensing instrumentation such as wind profilers (vertically pointing Doppler radars), the National Weather Service Doppler radar network, and lidars; and (3) special sensors deployed on board polar orbiting or geostationary satellites. The remotely sensed observations obtained from meteorological satellites have been especially helpful in providing crucial measurements of areally and vertically averaged temperature, moisture, and winds in data-sparse (mostly oceanic) regions of the world. Such measurements are necessary to accommodate modern numerical weather prediction practices and to enable forecasters to continuously monitor global storm (such as hurricane) activity. See also: Lidar; Meteorological instrumentation; Radar meteorology
At major operational weather prediction centers such as the National Center for Environmental Prediction (NCEP, formerly known as the National Meteorological Center or the European Centre for Medium Range Weather Forecasts, ECMWF), the global meteorological observations are routinely collected, quality-checked, and mapped for monitoring purposes by humans responsible for overseeing the forecast process. The basic observations collected by NCEP are also disseminated online by Internet Data Distribution (IDD) or in mapped form by digital facsimile (DIFAX) to prospective users in academia and the public and private sectors. At NCEP and ECMWF (and other centers) the global observational data stream is further machine-processed in order to prepare a full three-dimensional set of global surface and upper air analyses of selected meteorological fields at representative time periods. The typical horizontal and vertical resolution of the globally gridded analyses is about 100 km (60 mi) and 25–50 hectapascals (millibars), respectively. Preparation of these analyses requires a first-guess field from the previous numerical model forecast against which the updated observations are quality-checked and objectively analyzed to produce an updated global gridded set of meteorological analyses.
These updated analyses are modified as part of a numerical procedure designed to ensure that the gridded meteorological fields are dynamically consistent and suitable for direct computation in the new forecast cycle. This data assimilation, analysis, and initialization procedure, known as four-dimensional data assimilation, was at one time performed four times daily at the standard synoptic times of 0000, 0600, 1200, and 1800 UTC. The four-dimensional data assimilation is performed almost continuously, given that advanced observational technologies (such as wind profilers; automated surface observations; automated aircraft-measured temperature, moisture, and wind observations) have ensured the availability of observations at other than the standard synoptic times mentioned above. An example is the rapid update cycle (RUC) mesoscale analysis and forecast system used at NCEP to produce real-time surface and upper air analyses over the United States and vicinity every 3 h and short-range (out to 12 h) forecasts. The rapid update cycle system takes advantage of high-frequency data assimilation techniques to enable forecasters to monitor rapidly evolving mesoscale weather features.
Operational models
The forecast models used at NCEP and ECMWF and the other operational prediction centers are based upon the primitive equations that govern hydrodynamical and thermodynamical processes in the atmosphere. The closed set of equations consists of the three momentum equations (east-west, north-south, and the vertical direction), a thermodynamic equation, an equation of state, a continuity equation, and an equation describing the hydrological cycle. Physical processes such as the seasonal cycle in atmospheric radiation, solar and long-wave radiation, the diurnal heating cycle over land and water, surface heat, moisture and momentum fluxes, mixed-phase effects in clouds, latent heat release associated with stratiform and convective precipitation, and frictional effects are modeled explicitly or computed indirectly by means of parametrization techniques. A commonly used vertical coordinate in operational prediction models is the sigma coordinate, defined as the ratio of the pressure at any point in the atmosphere to the surface (station) pressure.
The NCEP operational global prediction model is known as the medium-range forecast (MRF) model. The medium-range forecast is run once daily out to 14 days from the 0000 UTC analysis and initialization cycle in support of medium-range and extended-range weather prediction activities at NCEP. The medium-range forecast run commences shortly before 0900 UTC in order to allow sufficient time for delayed 0000 UTC surface and upper air data to reach NCEP and to be included in the analysis and initialization cycle.
The aviation forecast model (AVN) is run from the 1200 UTC analysis and initialization cycle in support of NCEP's global aviation responsibilities. The aviation forecast model and medium-range forecast are identical models except that the AVN is run with a data cutoff of about 3 h and only out to 72 h in order to minimize the time necessary to get the model forecast information to the field. The MRF runs with a vertical resolution of 38 levels, a horizontal resolution of about 105 km (63 mi) [triangular truncation in spectral space] for the first eight days, and a degraded horizontal resolution of about 210 km (130 mi) for the last six days of the 14-day operational forecast.
The medium-range forecast is also the backbone of the ensemble forecasting effort (a series of parallel medium-range forecast runs with slightly changed initial conditions and medium-range forecast runs from different time periods) that was instituted in the early 1990s to provide forecaster guidance in support of medium-range forecasting (6–10-day) activities at NCEP. The scientific basis for ensemble prediction is that model forecasts for the medium and extended range should be considered stochastic rather than deterministic in nature in recognition of the very large forecast differences that can occur on these time scales between two model runs initialized with only very slight differences in initial conditions. Continuing advances in computer power have made it practical to begin operational ensemble numerical weather prediction. Given that each member of the ensemble (the ensemble consists of 38 different model runs) represents an equally likely model forecast outcome, the spread of the ensemble forecasts is taken as a measure of the potential skill of the forecasts. Forecasters look for clustering of the ensemble members around a particular solution as indicative of higher probability outcomes as part of a process inevitably known as “forecasting the forecasts.” Ensemble forecasting techniques are also taking hold at other operational centers such as the ECMWF, and they are likely to spread to regional models such as the NCEP Eta and Regional Analysis and Forecast (RAFS) models in the near future.
Regional forecast models such as the NCEP Eta model are utilized to help predict atmospheric circulation patterns associated with convective processes and terrain forcing. Given the increasing importance of forecasting significant mesoscale weather events (such as squall lines, flash flood occurrences, and heavy snow bands) that occur on time scales of a few hours and space scales of a few hundred kilometers, the Eta model will continue to be developed at NCEP in support of regional model guidance to local forecasters. An important challenge to mesoscale models is to make better precipitation forecasts, especially of significant convective weather events. Success in this endeavor will require increased use of satellite- and land-based data sets, especially measurements of water vapor, that are coming online.
Forecast products and forecast skill
These are classified as longer term (greater than two weeks) and shorter term.
Longer term
The Climate Prediction Center (CPC) of NCEP initiated a long-lead climate outlook program for the United States in early 1995. The scientific basis for this initiative rests upon the understanding of the coupled nature of global atmospheric and oceanic circulations on intraseasonal and interannual time scales. Particular attention has been focused on the climatic effects arising from low-frequency changes in global atmospheric wind, pressure, and moisture patterns associated with evolving tropical oceanic sea surface temperature and thermocline depth anomalies known as part of the El Niño–Southern Oscillation (ENSO) climate signal. Statistically significant temperature and moisture anomalies have been identified over portions of the conterminous United States (and in many other regions of the world) that can occur in association with an ENSO event, especially during the cooler half of the year. For example, in winter there is a tendency for the southern United States from Texas to the Carolinas, Georgia, and Florida to be wetter and cooler than normal, while much of the Pacific Northwest eastward across southern Canada and the northern United States to the Great Lakes is warmer and drier than normal during the warm phase (anomalies in sea surface temperature in the tropical Pacific from the Dateline eastward to South America are positive, while sea-level pressure anomalies are positive and negative, respectively, over the western versus central and eastern Pacific) of an ENSO event. See also: El Niño; Tropical meteorology
Similarly, the tropical convective activity that usually maximizes over the western Pacific oceanic warm pool (sea-surface temperature of about 30°C or 86°F) tends to shift eastward toward the central and, occasionally, eastern Pacific during the warm phase of an ENSO event. Under such conditions, drought and heat-wave conditions may prevail over portions of Australia and Indonesia, while conditions become much wetter in the normally arid coastal areas along western Peru and northern Chile. During the exceptionally strong ENSO event of 1982–1983, extreme climatic anomalies were observed at many tropical and midlatitude locations around the world. During the cold phase of ENSO (known as La Niña) when anomalies in the sea surface temperature in the tropical eastern Pacific are negative and the easterly trade winds are stronger than average, many of these global climatic anomalies may reverse sign, such as occurred during the 1988–1989 cold event. Increased knowledge and understanding of the global aspects of atmospheric and oceanic low-frequency phenomena have resulted from combined observational, theoretical, and numerical research investigations that in part were stimulated by the extraordinary warm ENSO event of 1982–1983. The knowledge is now being applied toward the preparation of long-lead seasonal forecasts at the Climate Prediction Center and elsewhere. See also: Drought
In the extended forecasting procedure of the Climate Prediction Center, a total of 26 probability anomaly maps are prepared (one each for temperature and precipitation for equally likely below-normal and above-normal categories) for each of 13 lead times from 0.5 to 12.5 months. The probabilistic format (begun in 1982 with the issuance of monthly outlooks of temperature and precipitation for the United States) and accompanying skill assessment of these long-lead forecasts allows sophisticated users in the public and private sectors to make better use of the outlooks for a variety of business and planning purposes. The long-lead climate outlooks are made from a combination of the following methods: (1) a statistical procedure known as canonical correlation analysis that relates prior anomalies of global sea surface temperatures, Northern Hemisphere 700-hPa (mbar) heights, and temperature and precipitation anomalies over the United States to subsequent anomalies; (2) another statistical procedure known as optimal climate normals that projects the persistence of recent temperature and precipitation anomalies to future months and seasons; and (3) forecasts of temperature and precipitation anomalies obtained from a coupled atmosphere-ocean general circulation model integrated forward in time six months by using selected sea surface temperature anomalies.
Shorter term
An important aspect of the forecasting process is the interpretation and dissemination of model forecasts to a wide variety of users and the general public. Specialized NCEP centers (in addition to the Climate Prediction Center) such as the National Severe Storms Forecasting Center (responsible for issuing watches and warnings of severe weather events), the Storm Prediction Center (charged with monitoring a broad range of winter and summer storms), the National Hurricane Center (responsible for monitoring the hurricane threat), and the Hydrological Meteorological Center (responsible for monitoring river basin water storage, runoff, and flood potential) are charged with providing guidance to local National Weather Service forecast offices about a variety of weather hazards. Experienced meteorologists and hydrologists at these centers are responsible for the interpretation of NCEP model and statistical forecasts so as to provide additional guidance and information on upcoming important weather events to individual offices of the National Weather Service. As an example, the Heavy Precipitation Branch of the NCEP has the responsibility of providing forecasts of significant rain and snow amount areas over the continental United States out to 48 h in advance. The experienced forecasters in the Heavy Precipitation Branch have been able to maintain their skill over the model objective precipitation forecasts since the mid-1970s, despite demonstrated model forecasting improvements, through a combination of detailed knowledge of regional and local mesometeorology, an understanding of systematic model biases and how to correct for them, and a continuing ability to select the best model on any given day in a form of human ensemble forecasting.
The skill of numerical weather prediction forecasts of temperature and winds in the free atmosphere [for example, the 500 hPa (mbar) level] continues to improve steadily. For example, since the mid-1980s the root-mean-square vector error of the 24-h 250-hPa (mbar) wind forecasts produced by the operational global NCEP model has declined from 10 m s−1 to 7.5 m s−1 (20 mi/h to 16 mi/h). Experience with medium-range-forecast/ECMWF day five predictions of mean sea-level pressure [500 hPa (mbar) heights] over North America indicates that standardized anomaly correlation coefficient scores (a standardized anomaly is the anomaly divided by the climatological standard deviation and is used to avoid overweighting anomalies at higher latitudes relative to lower latitudes) range from 30 to 65 (experience suggests a standardized score >20 has operational forecast value) with the higher (lower) scores occurring in winter (summer). At NCEP, the human forecasters have been able to improve upon the medium-range-forecast anomaly correlation coefficient scores for day five mean sea-level pressure forecasts.
Forecasts of surface weather elements such as temperature, precipitation type and amount, ceiling, and visibility are prepared objectively by a statistical-dynamical model output statistics (MOS) technique. The cornerstone of the MOS approach is the preparation of a series of regression equations for individual weather elements at specified locations, with the regression coefficients determined from predictors provided by operational NCEP models. MOS guidance is relayed to National Weather Service offices and a wide variety of external users. The MOS product has become very competitive with the human forecaster as measured by standard skill scores. Over the 25-year period ending in 1992, the mean absolute error of National Weather Service maximum and minimum temperatures issued to the general public decreased by about 0.6°C (1.08°F). To put this number in perspective, the skill of 36-h temperature forecasts issued in the late 1990s is comparable to the skill of similar 12-h forecasts made in the late 1960s. A similar “24-h gain” in skill level has been registered for routine 12-h probability-of-precipitation forecasts of the National Weather Service. Skill levels are a function of the forecast type and projection. Skill (as measured relative to a climatological control by using a standard skill measure such as the Brier score) in day-to-day temperature forecasting approaches zero by 7–8 days in advance. For probability-of-precipitation forecasts the zero skill level is typically reached 2–3 days earlier. Precipitation-amount forecasts typically show little skill beyond three days, while probability-of-thunderstorm forecasts may be skillful only out to 1–2 days ahead.
These varying skill levels reflect the fact that existing numerical prediction models such as the medium-range forecast have become very good at making large-scale circulation and temperature forecasts, but are less successful in making weather forecasts. An example is the prediction of precipitation amount and type given the occurrence of precipitation and convection. Each of these forecasts is progressively more difficult because of the increasing importance of mesoscale processes to the overall skill of the forecast. See also: Precipitation (meteorology)
Nowcasting
Nowcasting is a form of very short-range weather forecasting. The term nowcasting is sometimes used loosely to refer to any area-specific forecast for the period up to 12 h ahead that is based on very detailed observational data. However, nowcasting should probably be defined more restrictively as the detailed description of the current weather along with forecasts obtained by extrapolation up to about 2 h ahead. Useful extrapolation forecasts can be obtained for longer periods in many situations, but in some weather situations the accuracy of extrapolation forecasts diminishes quickly with time as a result of the development or decay of the weather systems.
Comparison with traditional forecasting
Much weather forecasting is based on the widely spaced observations of temperature, humidity, and wind obtained from a worldwide network of balloon-borne radiosondes. These data are used as input to numerical-dynamical weather prediction models in which the equations of motion, mass continuity, and thermodynamics are solved for large portions of the atmosphere. The resulting forecasts are general in nature. Although these general forecasts for one or more days ahead have improved in line with continuing developments of the numerical-dynamical models, there have not been corresponding improvements in local forecasts for the period up to 12 h ahead. The problem is that most of the mathematical models cope adequately with only the large weather systems, such as cyclones and anticyclones, referred to by meteorologists as synoptic-scale weather systems. See also: Weather
Nowcasting involves the use of very detailed and frequent meteorological observations, especially remote-sensing observations, to provide a precise description of the “now” situation from which very short-range forecasts can be obtained by extrapolation. Of particular value are the patterns of cloud, temperature, and humidity which can be obtained from geostationary satellites, and the fields of rainfall and wind measured by networks of ground-based radars. These kinds of observations enable the weather forecaster to keep track of smaller-scale events such as squall lines, fronts and thunderstorm clusters, and various terrain-induced phenomena such as land-sea breezes and mountain-valley winds. Meteorologists refer to these systems as mesoscale weather systems because their scale is intermediate between the large or synoptic-scale cyclones and the very small or microscale features such as boundary-layer turbulence. See also: Front; Meteorological satellites; Squall line; Storm
There is a distinction between traditional forecasting and nowcasting in terms of the lead time and quality of the forecast (Fig. 3). The two approaches are complementary; each has its place according to the lead time and detail required of the forecast. Conceptually, nowcasting is a simple procedure. However, vast amounts of data are involved, and it is only since the development of the necessary digital data-processing, transmission, and display facilities that it has been possible for nowcasting to be available economically. See also: Data communications
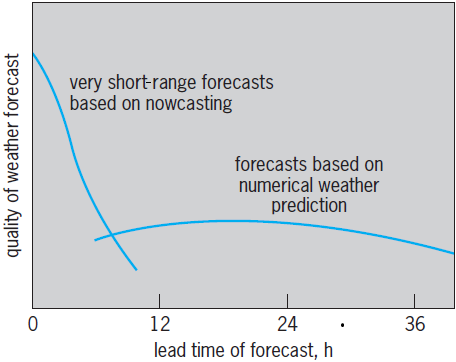
Information technology
A key problem in nowcasting is that of combining diverse and complex data streams, especially conventional meteorological data with remote-sensing data. It is widely held that this combination should be achieved by using digital data sets displayed on interactive video displays. By means of advanced human-computer interaction techniques, the weather forecaster will eventually be able to analyze the merged data sets by using a light pen or a finger on touch-sensitive television screens. Various automatic procedures can be implemented to help the forecaster carry out analyses and extrapolation forecasts, but the incomplete nature of the data sets is such that the forecaster will almost always be in the position of needing to fine-tune the products subjectively. The idea behind the forecasting workstation is to simplify the routine chores of basic data manipulation so that the forecaster is given the maximum opportunity to exercise judgment within the context of what is otherwise a highly automated system.
Very short-range forecast products are highly perishable: they must be disseminated promptly if they are not to lose their value. Advances in technology offer means for the rapid tailoring and dissemination of the digital forecast information and for presenting the material in convenient customer-oriented formats.
Nowcasting and mesoscale models
There is some evidence of a gap in forecasting capability between nowcasting and traditional synoptic-scale numerical weather prediction. This occurs for forecast lead times between about 6 and 12 h, that is, for periods when development and decay are beginning to invalidate forecasts by simple extrapolation (Fig. 3). To some extent the forecaster can identify some of the likely developments by interpreting the nowcast information in the light of local climatologies and conceptual life-cycle models of weather systems. But the best way to forecast changes is to use numerical-dynamical methods. It would be natural to assume that an immediate way would be to incorporate the detailed (but usually incomplete) nowcast data as input to numerical-dynamical models with a finer resolution than those presently in operational use; these are the so-called mesoscale numerical models. Research into the use of detailed observational data in mesoscale models has been actively pursued, but significant technical difficulties have slowed progress.
Extended-Range Prediction
Forecasts of time averages of atmospheric variables, for example, sea surface temperature, where the lead time for the prediction is more than two weeks, are termed long-range or extended-range climate predictions. The National Weather Service issues extended-range predictions of monthly and seasonal average temperature and precipitation near the middle of each month; these are known as climate outlooks. Monthly outlooks have a single lead time of two weeks. The seasonal outlooks have lead times ranging from two weeks to months in one-month increments. Thus, in each month a set consisting of one monthly and 13 seasonal long-lead-time climate outlooks is released.
Accuracy
The accuracy of long-range outlooks has always been modest because the predictions must encompass a large number of possible outcomes, while the observed single event against which the outlook is verified includes the noise created by the specific synoptic disturbances that actually occur and that are unpredictable on monthly and seasonal time scales. According to some estimates of potential predictability, the noise is generally larger than the signal in middle latitudes.
An empirical verification method called cross-validation is used to evaluate most statistical models. In cross-validation the model being evaluated is formulated by using all observations except for one or more years, one of which is then predicted by the model. This is done, in turn, for all the years for which observations are available. The observations for each of the years withheld are then compared with their associated outlooks and the forecast accuracy is determined.
For coupled model forecasts, cross-validation is not used. Rather, the model must be rerun and verified for at least 10 years (cases) for a given season. These coupled model reruns, based upon observed sea surface temperatures, indicate that these forecasts of temperature have a cold-season United States average maximum correlation with observations of about 0.50 and a warm-season minimum score near zero. The average maximum score is reduced by about half when strong El Niño–Southern Oscillation (ENSO) years are excluded. Coupled model precipitation forecasts over the United States have early winter and early spring accuracy maxima (correlations with observations of 0.30–0.40) when ENSO cases are included. Correlations between forecast and observed precipitation fields for this parameter also decline to about 0.10–0.15 when strong ENSO cases are excluded. See also: El Niño
When forecasts of seasonal mean temperature made using the canonical correlation technique are correlated with observations, accuracy maxima in winter and summer and minima in spring and autumn are obtained. The score at lead times of one, four, and seven months is nearly the same. Only at lead times of 10 and 13 months does the correlation drop to about half its value at the shorter lead times. As measured by the correlation between forecasts and observations, temperature forecasts made by the optimal climate normals technique have summer and winter maxima and minima in late winter–early spring and autumn. One interesting consequence of these temperature outlook accuracy properties is that, at certain times of the year, short-lead predictions may have lower expected accuracy than much longer lead predictions.
The accuracy of precipitation outlooks is generally lower than that of temperature outlooks. Precipitation predictions of the canonical correlation analysis and the optimal climate normals techniques have wintertime maximum correlations with observations. However, the former has low summertime accuracy, while the latter has relatively high accuracy during that season. For both methods, areas of high expected accuracy on the precipitation maps are much smaller and more spotty than those for temperature. This is due to the fact that precipitation is much more noisy (that is, variable on small space and time scales) than temperature.
Forecasts of seasonal mean temperature made by canonical correlation analysis and optimal climate normals have average correlations with observations in excess of 0.5 over large portions of the eastern United States during January through March and over the Great Basin during July through September.
Formulating an outlook
Forecasts of monthly and seasonal mean surface temperature and total precipitation, for the conterminous United States, Alaska, and Hawaii, are derived from a quasiobjective combination of canonical correlation analysis, optimal climate normals, and the coupled ocean-atmosphere model. For each method, maps consisting of standardized forecast values at stations are produced by computer. The physical size of the plotted numbers indicates the accuracy of the model. Two forecasters examine the map or maps for each lead time and variable, and resolve any conflicts among the methods. The statistical confidence in the resulting forecast will be low in regions where two or more equally reliable tools are in opposition or where the expected accuracy is low for all methods. Confidence is relatively high when tools agree and when the expected accuracy is high.
Information about accuracy and confidence is quantified through the use of probability anomalies to indicate the degree of confidence available from the tools. The climatological probabilities, with respect to which the outlook anomalies deviate, are determined well ahead of time. This is done by first dividing the observations of seasonal mean temperature and total precipitation during 1961–1990 into three equally likely categories for each of the stations at which forecasts are made. These are below, normal, and above for temperature, and below, moderate, and above for precipitation. The likelihood of these categories is and is designated CL (for climatological probabilities) on the maps. Regions in which the correlation between the forecasts made by the tools and the observations, as determined by cross-validation, is less than 0.30 are given this designation. Elsewhere, nonzero probability anomalies—the numerical size of which is based upon the correlation scores of the tools, the degree of agreement among the tools, and the amplitude of the composite predicted anomalies—are assigned. Finally, the forecasters sketch the probability anomaly maps. See also: Weather map